Cybertherapy: Clinician “Extenders” and 3D Virtual Health Assistants
The following featured projects describe some of the milestones we reached, with evolving promising results, toward designing and implementing effective, supportive, and enjoyable interactions with personalized virtual health assistants, or clinician “extenders” that can help people with their health and well-being. Articles on these and other projects are listed on our lab Publication page.
Delivering a Behavior Change Intervention with an Empathic Embodied Virtual Agent
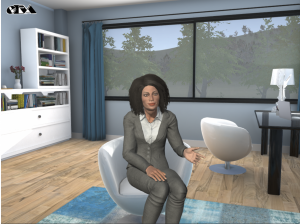
One eEVA agent situated in her 3-D office (click on image to view demo).
We used the eEVA framework (described below) to build a virtual health agent able to deliver an evidence-based (i.e. considered effective based on scientific evidence with controlled experiments) Brief Motivational Interviewing intervention (BMI). BMIs helps people find intrinsic motivation to change lifestyle issues placing them at-risk of serious illness (e.g. heavy alcohol consumption, drug use, overeating), using empathic motivational interviewing.
We developed a model of agent’s nonverbal behaviors for that is appropriate for therapy by analyzing a video corpus of therapy sessions delivered by a human counselor. This eEVA agent delivers a BMI targeted at people with heavy alcohol consumption, by talking and understanding user’s spoken answers, and displaying therapy-appropriate facial expression and gestures.
Results of the evaluation of the feasibility, acceptability, and utility of the eEVA intervention with heavy drinkers recruited over the Internet, show that participants had overwhelmingly positive experiences with the digital health agent, including engagement with the technology, acceptance, perceived utility and intent to use the technology (Boustani, et al., 2021).
Embodied Empathic Virtual Agents (eEVA) Framework
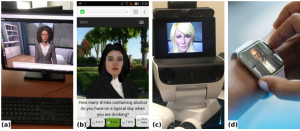
eEVA running on different platforms: (a) desktop, (b) mobile phone (click on image to view demo), (c) autonomous robot, (d) smartwatch concept.
We designed and developed the Embodied Empathic Virtual Agents (eEVA) framework to enable the modular construction of dialog-based interactions with an expressive 3-dimensional full-body animated virtual agent, and so, in realtime, over the Internet, and on different platforms (e.g. PC, mobile phones, robots, watches). See video by clicking on figure.
Our objective performance tests (Polceanu and Lisetti, 2019) revealed that it is possible for a 3-dimensional agent evolving in a 3-dimensional office (i.e. computationally intensive) to reliably interact with users in realtime, using speech recognition of the user’s read answers, speech synthesis of the agent’s utterances, nonverbal full-body 3-dimensional animations and realistic facial expressions, and other traditional interaction modalities (e.g. buttons, menus, text entries).
Unconstrained Spoken Dialog with a Virtual Health Agent
We focused on designing our virtual health agent so that it can deliver a brief motivational intervention (BMI, see above) using not only speech recognition, but also dialog management to enable the user to speak and answer questions freely, i.e. without restricting them to read from pre-set provided answers.
Unlike chatbots – that do not track where they are in a conversation – our virtual health assistant can carry out a conversation, knows which question(s) it successfully receives an answer to, rephrases a question if it did not get an answer to, and asks for confirmation when it is unsure of the user’s answers.
Using data we collected from real user interactions and reinforcement learning, the dialog manager begins to learn optimal dialog strategies for initiative selection and for the type of confirmations used during the interaction. We then compared the unoptimized system with the optimized system in terms of objective measures (e.g. task completion) and subjective measures (e.g. ease of use, future intention to use the system) with positive results.
Publications:
- C. Lisetti, A. Amini, and U. Yasavur (2015). Now All Together: Overview of Virtual Health Assistants Emulating Face-to-Face Health Interview Experience. Künstliche Intelligenz (DOI 10.1007/s13218-015-0357-0).
- U. Yasavur, C. Lisetti and N. Rishe (2014). Let’s talk! A Speaking Virtual Counselor Offers you a Brief Intervention. Journal on Multimodal User Interfaces. Vol 8, pp 381–398.
- U. Yasavur, and C. L. Lisetti, and N. Rishe (2013). Modeling Brief Alcohol Intervention Dialogue with MDPs for Delivery by ECAs. In Proceedings of the 13th International Conference on Intelligent Virtual Agents (IVA’13) (Edinburgh, SCOTLAND, August 2013).
On-Demand Virtual Counselor (ODVIC) Mirrors the User’s Social Cues
We investigated the impact of endowing embodied conversational agents with the ability to mirror the user’s facial expressions, and to portray other socially appropriate nonverbal behaviors (NVB) (e.g. head and hand movements), based on the content of the conversation.
Using our HapFACS avatar system, we built the On-Demand VIrtual Counselor (ODVIC) system (Lisetti et al., 2013), which is controlled by a multimodal Embodied Conversational Agent (ECA) that empathically delivers a brief motivational intervention found effective by clinicians with funding from the National Institute of Health (click on image to see video).
We developed two models of non-verbal behaviors, which we integrated in ODVIC: one system is rule-based, and the other uses machine learning.
Our first study using the rule-based model of non-verbal behaviors shows a 31% increase in users’ intention to reuse the intervention when the content of the intervention is delivered by our ODVIC, compared to users’ intention to reuse the same intervention delivered with a text-only user interface (Lisetti et al., 2013).
A second study uses a data-driven approach to learn from a human counselor’s nonverbal behaviors (head nods, facial expressions, hand movements) during a session with a client , recorded and annotated for machine learning (Amini, Boustani, and Lisetti, 2021).
Results show high accuracy of the individual models and improvements of the rapport-enabled ECA over a neutral ECA, for measures of rapport, attitude, intention to use, perceived enjoyment, perceived ease of use, perceived sociability, perceived usefulness, social presence, trust, likability, and perceived intelligence.
Ontology for Behavioral Health
Named-Entity Recognizers (NERs) are an important part of information extraction systems in annotation tasks. Although substantial progress has been made in recognizing domain-independent named entities (e.g. location, organization and person), there is a need to recognize named entities for domain-specific applications in order to extract relevant concepts.
We have developed the first named-entity recognizer designed for the lifestyle change domain. It aims at enabling smart health applications to recognize relevant concepts. We created the first ontology for behavioral health (shown below) based on which we developed an NER augmented with lexical resources. Our NER automatically tags words and phrases in sentences with relevant (lifestyle) domain-specific tags. For example, it can tag: healthy food, unhealthy food, potentially-risky, healthy activity, drugs, tobacco, alcoholic beverages.
Exposure Therapy for Anxiety Disorders in Virtual Environments
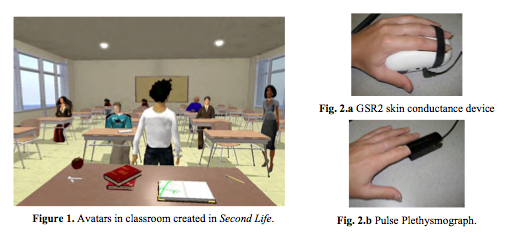
Avatars in classroom created in Second Life.
We designed a study to help people with anxiety disorders via exposure therapy by eliciting anxiety-producing emotionally loaded stimuli in 3-dimensional virtual environment, while measuring their physiological signals (galvanic skin response (GSR) and heart rate variability (HRV) using biosensors.
Exposure therapy is an evidence-based (i.e. considered effective based on scientific evidence with controlled experiments) psychotherapeutic intervention that exposes the patient to feared situations and objects, found to be effective in the reduction of phobic and anxious symptomatology (e.g. phobias, anxiety disorder, Post-Traumatic Stress Disorder (PTSD)). The principle is to slowly increase the level of intensity of the feared stimuli over multiple sessions, with the goal to slowly desensitize the patient to the stimuli by developing strong expectations of successful outcomes in the recreated situation (e.g. the student delivers successful oral presentations).